Revolutionizing Business with Data Labeling in Machine Learning
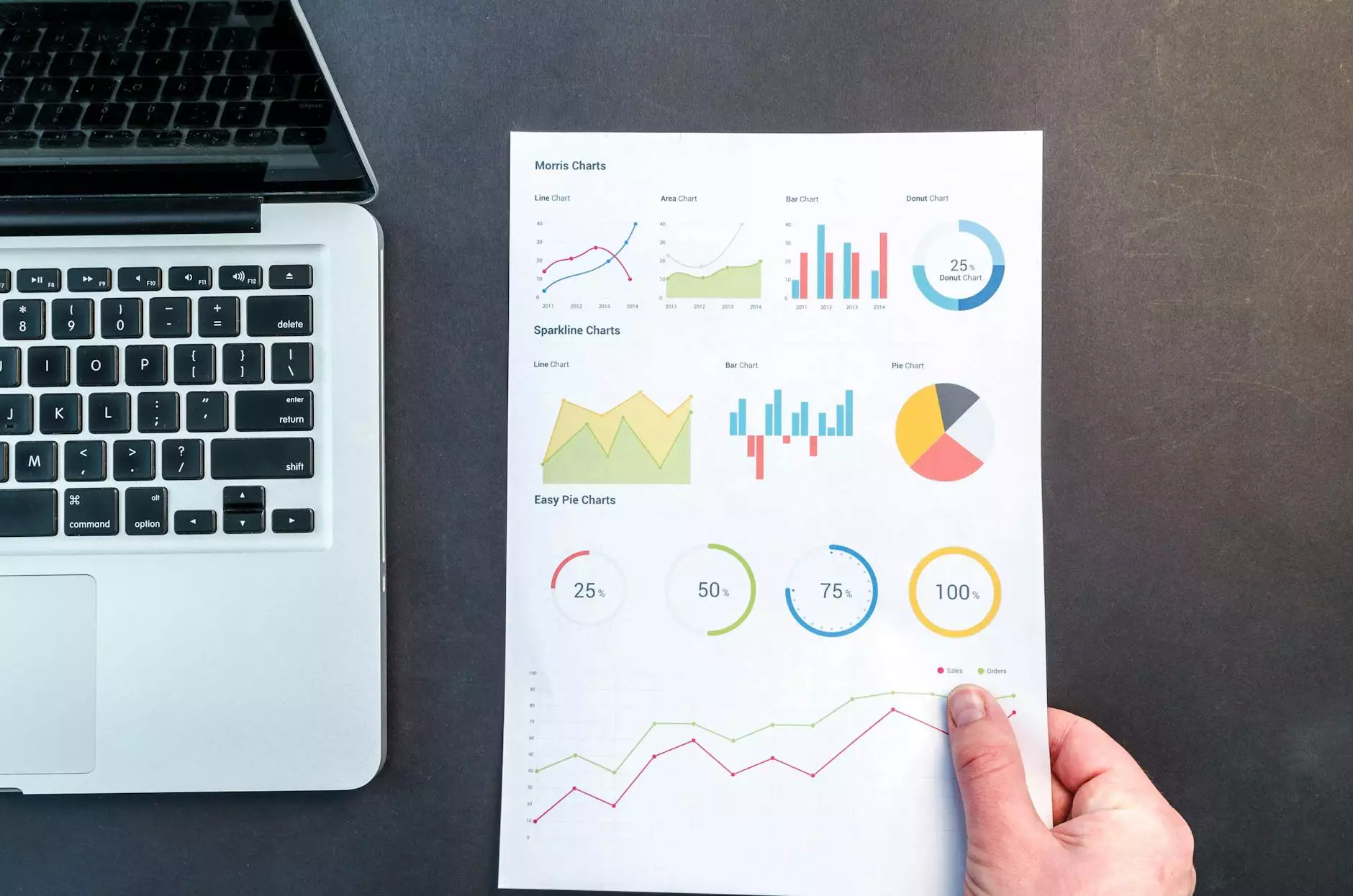
Understanding the Importance of Data Labeling
In the realm of machine learning, the success of algorithms heavily relies on the quality of the data fed into them. Data labeling plays a pivotal role in ensuring that machine learning models can learn accurately and efficiently. By categorizing and tagging datasets, businesses can enhance their models' performance and achieve a competitive edge in the market.
The Process of Data Labeling
Data labeling involves the process of identifying raw data and adding informative tags or labels to it. This task can be performed manually by human annotators or through automated systems. The choice of method depends on several factors, including data volume, complexity, and required accuracy. Here's how the process typically unfolds:
- Data Collection: Gathering relevant data from various sources such as customer interactions, surveys, or IoT devices.
- Annotation: Manually or automatically labeling the collected data, which might include text, images, videos, or audio.
- Verification: Ensuring the accuracy and consistency of the labels through quality checks.
- Integration: Feeding the labeled data into machine learning algorithms for training.
Key Benefits of Data Labeling for Businesses
The integration of data labeling techniques can provide numerous benefits, particularly for businesses in the Home Services and Keys & Locksmiths sectors. Here are some of the key advantages:
- Enhanced Accuracy: Labeled data enables machine learning models to make more precise predictions, thus improving service quality.
- Increased Efficiency: Automating tasks such as service requests or inventory management can streamline operations.
- Better Customer Insights: Analyzing labeled data helps businesses understand customer preferences and behaviors, facilitating tailored services.
- Scalability: Well-labeled data can easily be scaled to accommodate growing data sizes as businesses expand.
Applications of Data Labeling in Home Services
In the Home Services industry, data labeling facilitates various applications. For instance, companies can leverage labeled datasets for predictive maintenance, where machine learning models can predict potential service needs based on historical data. Here are some specific applications:
- Service Allocation: Assigning technicians to service requests based on past performance metrics, improving response time.
- Customer Behavior Prediction: Understanding the likelihood of customers requesting service based on seasonality and past behavior.
- Feedback Analysis: Automating the analysis of customer feedback to identify areas for improvement.
Data Labeling in the Locksmith Industry
For the Keys & Locksmiths sector, data labeling can revolutionize the way business operations are managed. Machine learning models can analyze trends and optimize inventory based on labeled historical data. Consider the following applications:
- Emergency Response Optimization: Predicting high-demand periods for locksmith services, enabling better resource allocation.
- Inventory Management: Using labeled data to predict which products will be in demand, minimizing overstock and stockouts.
- Customer Service Enhancement: Automatically categorizing feedback to ensure timely responses and improved service delivery.
The Role of Technology in Data Labeling
The evolution of technology has significantly impacted data labeling practices. The introduction of advanced tools like machine learning platforms, annotation software, and automation solutions has made the data labeling process more efficient. Businesses can now leverage these technologies to enhance their machine learning initiatives:
- Crowdsourcing Platforms: Utilization of these platforms allows for quick, large-scale data labeling, increasing both speed and efficiency.
- Automated Annotation Tools: Using AI-driven tools to pre-label data, which human annotators can then verify, significantly reduces workload.
- Data Management Systems: Centralized systems ensure organized storage and management of datasets, making data retrieval for labeling faster and easier.
Challenges in Data Labeling
While data labeling offers immense benefits, it is not without challenges. Organizations must be aware of potential hurdles:
- Quality Control: Maintaining the accuracy and consistency of labels can be challenging, especially with large datasets.
- Resource Allocation: Labeling tasks can be time-consuming and may require additional manpower.
- Data Privacy Concerns: Ensuring compliance with data protection regulations while labeling sensitive information is critical.
Best Practices for Effective Data Labeling
To maximize the benefits of data labeling, businesses should adopt best practices:
- Define Clear Guidelines: Establish comprehensive labeling instructions to ensure consistency among annotators.
- Invest in Quality Assurance: Implement regular audits and validation checks to monitor labeling accuracy.
- Leverage Collaboration Tools: Use tools that allow for seamless communication among team members involved in the labeling process.
- Continuous Training: Provide ongoing training for annotators to keep them updated on best practices and industry standards.
Conclusion
In conclusion, data labeling is a fundamental aspect that significantly influences the effectiveness of machine learning models. For businesses in Home Services and Keys & Locksmiths, the ability to leverage labeled data can lead to improved service quality, operational efficiency, and enhanced customer relationships. By embracing innovative data labeling techniques and adhering to best practices, organizations can achieve sustainable growth and a competitive advantage in their markets.
With the ongoing advancements in technology and the increasing importance of data-driven decision-making, investing in robust data labeling strategies is essential for any business aiming to thrive in today’s data-centric environment.
data labeling machine learning