The Comprehensive Guide to Image Classification Labeling Tools
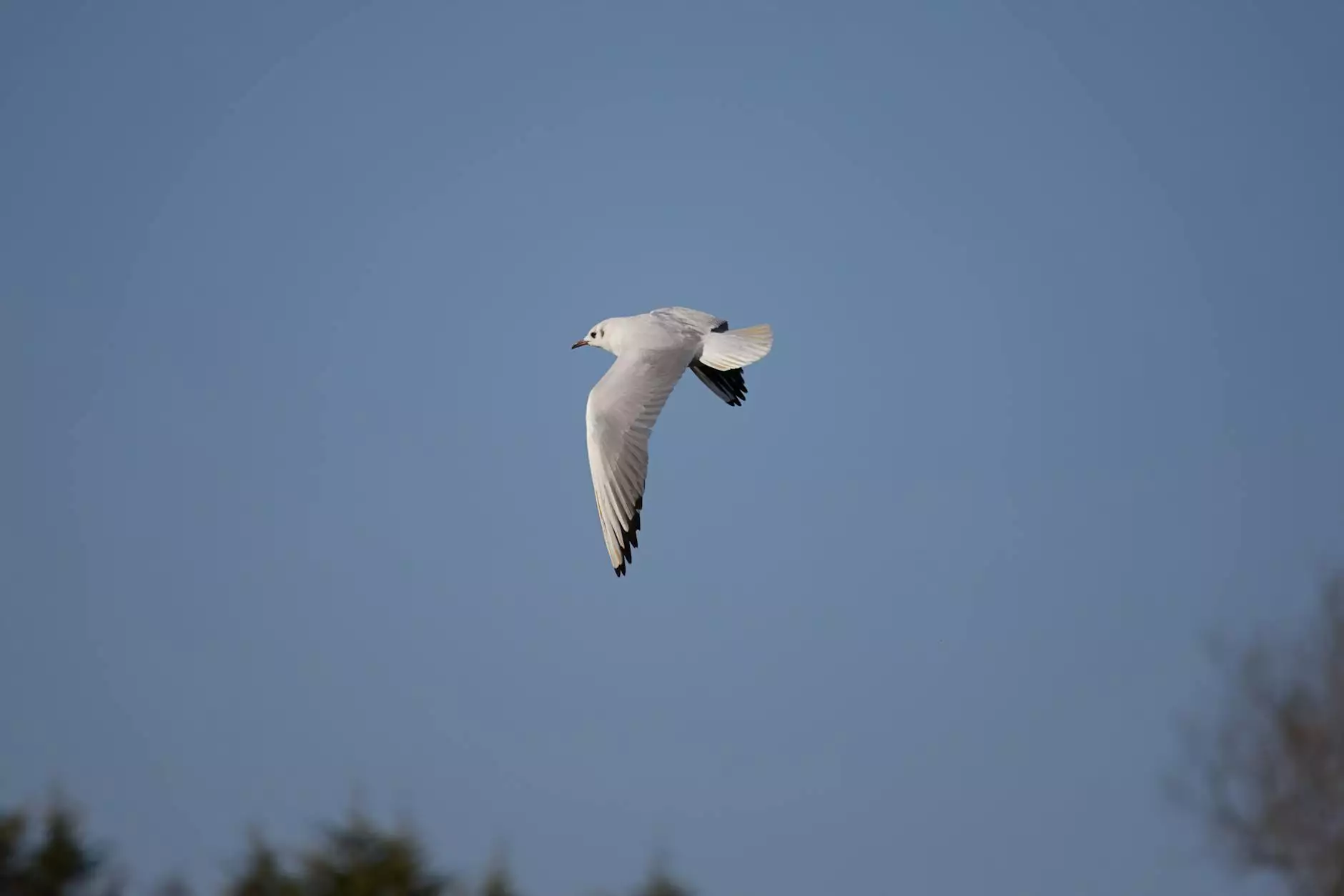
In today's digital landscape, the importance of image classification cannot be overstated. From machine learning to artificial intelligence applications, precise labeling of images is critical. This article delves into the intricacies of image classification labeling tools, their role in software development, and how they help businesses achieve unparalleled efficiency and accuracy in their projects.
Understanding Image Classification
Image classification is a process wherein algorithms analyze visual data and categorize it into predefined classes. This categorization is foundational for various applications including image recognition, autonomous vehicles, and medical imaging. However, to train these algorithms effectively, a significant amount of annotated images is required. This is where image classification labeling tools come into play.
The Role of Labeling Tools in Software Development
Labeling tools simplify the intricate task of annotating images by providing a user-friendly interface for data scientists and developers. Here are key benefits of using image classification labeling tools in software development:
- Enhanced Accuracy: Tools facilitate detailed and consistent labeling, reducing human error.
- Increased Efficiency: Automated processes can handle large datasets far quicker than manual labeling.
- Scalability: As a project grows, labeling tools can adapt to handle increased workloads without compromising quality.
- Cost-Effectiveness: Reducing the time spent on annotation directly translates to lower operational costs.
How Image Classification Labeling Tools Work
Image classification labeling tools generally offer a suite of features aimed at streamlining the annotation process. Below is an outline of how these tools function:
1. Image Uploading
Users can easily upload images or entire datasets directly into the tool. Many labeling tools support various image formats, ensuring compatibility with your data.
2. Annotation Methods
These tools provide various annotation options, such as:
- Bounding Boxes: Drawing boxes around objects of interest.
- Polygonal Segmentation: Defining complex shapes for precise mapping of outlines.
- Point Annotation: Marking keypoints for specific features within images.
3. Quality Assurance
Many advanced image classification labeling tools include built-in quality assurance features that help ensure annotations meet defined standards. This might involve:
- Review Systems: Allowing team members to review and edit annotations before final submission.
- Model Feedback: Implementing feedback loops where model performance improves based on correct labels.
Choosing the Right Image Classification Labeling Tool
When selecting an image classification labeling tool, consider the following factors to ensure it meets your project needs:
- User Interface: Look for tools with intuitive interfaces that minimize the learning curve.
- Collaboration Features: Ensure the tool supports team-based workflows for larger projects.
- Integration Capability: Check if the tool can integrate with existing software and data pipelines.
- Cost: Assess the pricing model to find a solution that fits within your budget while meeting your quality requirements.
Impact on Machine Learning and AI
Image classification labeling tools are vital for the advancement of machine learning and artificial intelligence. The accuracy of models in these fields is heavily reliant on the quality of input data. Here’s how labeling tools contribute:
1. High-Quality Training Data
Machine learning algorithms learn from examples. The more accurately labeled the images are, the better the algorithm can perform. Poorly labeled data can result in biased models that fail to generalize.
2. Accelerated Model Training
With efficient labeling, datasets can be prepared quickly, allowing developers to train models more rapidly. This speed is critical in competitive industries where being first to market can provide a significant advantage.
3. Real-World Applications
From healthcare, where image classification tools are used for diagnostic purposes, to autonomous driving, image classification labeling tools have revolutionized the way industries operate. The correct labeling ensures that algorithms can make informed decisions based on visual data.
Case Studies: Real-World Applications of Image Classification Labeling Tools
Let’s explore some successful implementations of image classification labeling tools in various industries:
1. Healthcare
In the medical field, tools such as virtual microscope image analysis programs have leveraged image classification labeling to train algorithms capable of diagnosing conditions from histopathology images, significantly reducing time and increasing diagnostic accuracy.
2. Retail
Retailers have utilized image classification to enhance customer experiences. By employing intelligent classification tools, retailers can automate product categorization, improving search functionality and inventory management.
3. Autonomous Vehicles
Self-driving car companies use image classification labeling tools to annotate road signs, pedestrians, and obstacles, critical for training models that navigate complex environments safely.
Future Trends in Image Classification Labeling Tools
The landscape of image classification labeling tools is continuously evolving. Here are some future trends to keep an eye on:
- AI-Assisted Labeling: The integration of AI and machine learning into labeling tools is set to revolutionize accuracy by providing suggestions based on previously labeled data.
- Increased Automation: As AI continues to advance, expect labeling tools to automate more of the tedious annotation tasks.
- Enhanced Collaborative Features: Future tools will likely have improved collaboration features allowing teams to work more effectively across various geographical locations.
The Importance of Continuous Improvement
As technology evolves, so too must the tools we use. Continuous investment in the best image classification labeling tools not only optimizes current workflows but also prepares businesses for future challenges in image classification. Regular updates and adaptation will ensure your business remains competitive and efficient.
Conclusion
The relevance of image classification labeling tools in software development reflects the growing demand for accurate and efficient data annotation in the age of big data. Companies that harness these tools can streamline their development processes, enhance the performance of their machine learning models, and ultimately achieve a competitive edge in their respective fields.
As industries continue to rely heavily on visual data, investing in a robust image classification labeling tool will be pivotal in driving innovation and success. By choosing the right tool, organizations can ensure their initiatives are not only more effective but also future-proof in a rapidly advancing technological landscape.